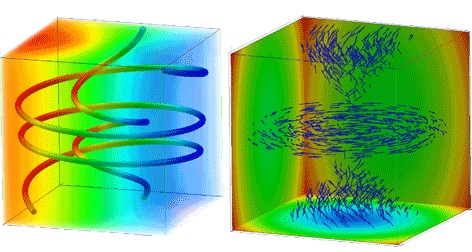
Support vectors learning for vector field reconstruction
Marcos Lage, Rener Castro, Fabiano Petronetto, Alex Bordignon, Geovan Tavares, Thomas Lewiner, Hélio Lopes
Sibgrapi 2009 (XXII Brazilian Symposium on Computer Graphics and Image Processing): pp. 104-111 (october 2009)
Abstract:
Sampled vector fields generally appear as measurements of real phenomena. They can be obtained by the use of a Particle Image Velocimetry acquisition device, or as the result of a physical simulation, such as a fluid flow simulation, among many examples. This paper proposes to formulate the unstructured vector field reconstruction and approximation through Machine-Learning. The machine learns from the samples a global vector field estimation function that could be evaluated at arbitrary points from the whole domain. Using an adaptation of the Support Vector Regression method for multi-scale analysis, the proposed method provides a global, analytical expression for the reconstructed vector field through an efficient non-linear optimization. Experiments on artificial and real data show a statistically robust behavior of the proposed technique.Downloads:
PDF paper (21.9 MB)PPT presentation (17.6 MB)
Movies of the PPT presentation (8.8 MB)
movie (23.1 MB)
YouTube
BibTeX:
@inproceedings{vectorfieldsvr_sibgrapi,author = {Marcos Lage and Rener Castro and Fabiano Petronetto and Alex Bordignon and Geovan Tavares and Thomas Lewiner and Hélio Lopes},
title = {Support vectors learning for vector field reconstruction},
year = {2009},
month = {october},
booktitle = {Sibgrapi 2009 (XXII Brazilian Symposium on Computer Graphics and Image Processing)},
pages = {104--111},
publisher = {IEEE},
address = {Rio de Janeiro, RJ},
doi = {10.1109/Sibgrapi.2009.20},
url = {\url{http://thomas.lewiner.org/pdfs/vectorfieldsvr_sibgrapi.pdf}}
}