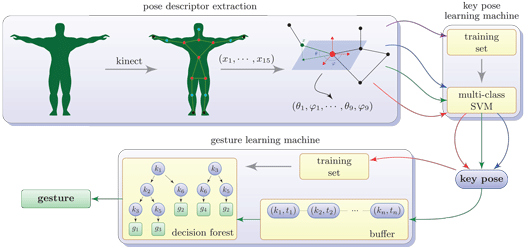
Online gesture recognition from pose kernel learning and decision forests
Leandro Miranda, Thales Vieira, Dimas Martinez, Thomas Lewiner, Antônio Wilson Vieira, Mario F. M. Campos
Pattern Recognition Letters 39: pp. 65-73 (april 2014)
Selected for publication from the Sibgrapi 2013 conference
Selected for publication from the Sibgrapi 2013 conference
Abstract:
The recent popularization of real time depth sensors has diversified the potential applications of online gesture recognition to end-user natural user interface (NUI). This requires significant robustness of the gesture recognition to cope with the noisy data from the popular depth sensor, while the quality of the final NUI heavily depends on the recognition execution speed. This work introduces a method for real-time gesture recognition from a noisy skeleton stream, such as those extracted from Kinect depth sensors. Each pose is described using an angular representation of the skeleton joints. Those descriptors serve to identify key poses through a Support Vector Machine multi-class classifier, with a tailored pose kernel. The gesture is labeled on-the-fly from the key pose sequence with a decision forest, which naturally performs the gesture time control/warping and avoids the requirement for an initial or neutral pose. The proposed method runs in real time and its robustness is evaluated in several experiments.Downloads:
BibTeX:
@article{gesture_learning_patrec,author = {Leandro Miranda and Thales Vieira and Dimas Martinez and Thomas Lewiner and Antônio Wilson Vieira and Mario F. M. Campos},
title = {Online gesture recognition from pose kernel learning and decision forests},
year = {2014},
month = {april},
journal = {Pattern Recognition Letters},
volume = {39},
pages = {65--73},
publisher = {Elsevier},
doi = {10.1016/j.patrec.2013.10.005},
url = {\url{http://thomas.lewiner.org/pdfs/gesture_learning_patrec.pdf}}
}